Transparent Model Reporting
Transparent Model Reporting is a guided, structred , standardized, analytical pathway for ML4H quality assurance/ model reporting practice / auditing framework
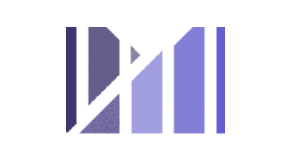
Scope
Transparent model reporting is research about one of the most critical topics about Machine Learning and Health: transparency.
When a researcher, laboratory, or company discovers a new technology or method to evaluate one topic, there is a standard behavior not to publish data or the codes, preserving as a secret object to the owner.
AI Audit doesn’t have the objective to publish somebody’s codes or data but creates trustworthiness between the users and providers. A study on the practice of transparent model reporting.
If you have a use case, feel free to submit us and receive an AI Audit analysis with total safety and expand the trustworthiness of your company.
Send an e-mail to [tmr.daisam.fgai4h@aiaudit.org].
Aims
- Create a discussion about transparency and trustworthiness between the users and developers
- Promotes more transparency between companies and users.
- Paper and regulatory documents to manufacturers, academics, health users notified regulatory bodies.
- Scientific publications, seminars & webinars.
Outputs
Collaboration resources
You are welcome to inquire about the work stream and opporunities for collaboration directly with the work stream team.
- General contact Jana Fehr, jana@aiaudit.org
Meetings
Regular meetings for this work stream take place at the below coordinates.
- Meetings Every Thursday, 4:00 PM, CET
- Zoom room Click here to join meeting
Communication
You can subsbscribe to the work stream mailing list to receive updates and join the asynchronous group chat.
- Group chat https://discord.gg/53zWbgxt
- Mailing list
Tools
We use different tools in our remote work. They include shared documents, github projects for code as well as task tracking and a collaborative whiteboard for ideation. You can request access via the below links.
- Shared drive
- Github project
- Collaborative whiteboard
You can find more information about the way we usually carry out our work remotely in teams here.
Milestones
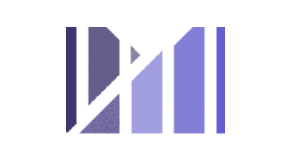
Important reference material
This is a list of related work and resources relevant for this work stream. It comprises resources the work stream contributors consider good practice.
Advertisement
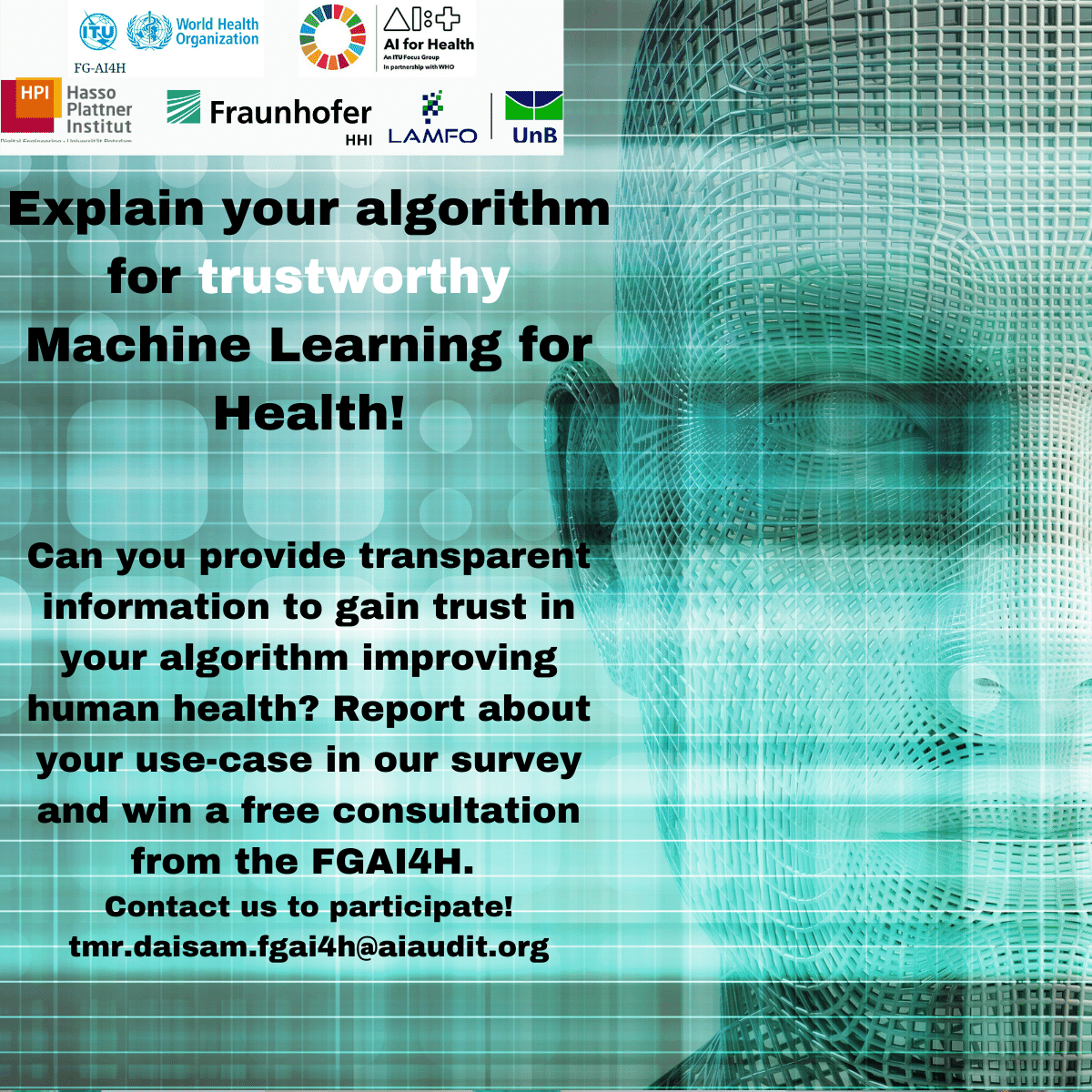